Sunday, April 15, 2007
Maps, Earth, NASA code...
Now I'm trying to decide what to use for my next little project, Google Earth, Google Maps, Yahoo Maps or maybe NASA North Wind. I'll be creating a photo-map-story-blog, showing photos I take with my new camera in different cities and with different stories to them....
Thursday, December 28, 2006
Reconstruction improvement
I have come to terms with a basic failing in the application, which doesn't reconstruct nearly as well as it should. I believe I know two additions which could fix the algorithm, but with less than a week left, it's not realistic to believe in such a solution. The additions would be:
- The addition of a point correspondence correction algorithm (by the original authors dubbed "the optimal solution"). This would correct clicked image points depending on epipolar constraints, which in turn would benefit the triangulation of 3D points.
- Iteration in the algorithm. After reconstructing a set of 3D coordinates, these should be tested by projection back into the image frame - if the results of this re-projection are inaccurate, make a new estimate of the camera pose, which is then used for a new triangulation. After all, the first pose estimation is only done with four manually defined image points, and each point after that is also clicked manually, of course leading to a great deal of error in the reconstruction. Perhaps a similar iteration could be applied to the calculation of the fundamental matrix, the algebraic representation of epipolar geometry.
Sunday, December 17, 2006
3D Reconstruction from photos and Image noise
The reconstruction done without enforcing this constraint is quite lousy, to say the least. Instead of searching through the software I created for errors (I've created quicker code, but not found any errors after using several methods of searching) I have decided to use 2-3 days for implementing a much more advanced algorithm, which enforces the epipolar constraint, and thereby "fixes" the problem of image noise...
If you're interested, an article named "Triangulation" from Hartley and Sturm, published in 1997 (sorry if any detail is wrong, no ill intention).
Hope this post helps you,
E.Hunefalk [First name not included because of spam risk - with some luck my thesis will published in 2007 though, so the name shouldn't be difficult to find :-)]
Wednesday, October 11, 2006
Modelling application
The shortest summary I've been able to create works as follows [extreme draft, but comments are still welcome]:
Modelling
The modelling section of the application consists of three subparts. These are called Pre-modelling, Parameter Value Generation and Post-modelling. In short, the system lets a user create models from his or her perception of the object of interest, followed by the step where images are used to find distances between different coordinates in the object and finally the user goes into the post-modelling part of the system, to correct possible mistakes made in the previous parts of the process.
Pre-modelling
Here, a user can specify how a building, or object (hereafter all specified as buildings), is put together. This is done by fitting different ‘blocks’ together, where a block could for example be a cube to model the base of the building or a pyramid for the roof. In this part, a user can specify parameter constraints, both in the same block and between different block. For example, the height is often the same at the four corners of a house, while the roof is aligned in all four directions of a square building, and also has its bottom at the top of the base.
The camera can be moved to fit background images, or the user can simply model on-the-fly.
Generating World Coordinate Values
In this part of the process, the user specifies 2D image coordinates – most often in photos – which correspond to 3D world coordinates for the final model. This is the most work intensive part of the process, and consists of three steps:
1) Find the Fundamental matrix (F) between images. By clicking on at least seven corresponding points the minimal solution, RANSAC or the eight-point algorithm can be used. Together with the cameras intrinsic parameters K and K’ the Essential matrix (E) can then be found. Through SVD the camera rotation and translation are derived.
2) For each point correspondence compute/triangulate the corresponding 3D space coordinate X that project to those image points. Initialize structure from two views. For each new image/image pair:
a. Determine pose
b. Refine current values (see more on point 3)
c. Extend structure
3) Weigh points depending on angle compared to camera – Better angle (width etc.) when straight forward than at narrow angle. All surfaces are two dimensional, and should be evaluated as such before moving to the third dimension. Pick corners in post-modelling stage by putting boxes (primitives) at each vertex. When vertices are the same for multiple surfaces, only use one box (which can be picked to change values).
4) Move coordinates to set one model corner at world origin (must stand on ground, with one corner at (0,0,0)). Show with a ground plane, and let user change coordinates. This way, we’ll align this corner with the tracked points from a video stream, where one corner should be set to (0,0,0) and the width/length of the tracked square should be set to the width/length of the modelled building.
5) Save mesh: Save the model as x-file, move texture images to assigned folder together with ".x"-files
With all these parameters, the application calculates 3D world coordinates depending on which coordinates have been specified from images, which different parameters should have the same value and if one or other value is unlikely for the world coordinate.
Post-modelling
This part is very similar to the pre-modelling. Here, the user can look at the values given from the second step of the modelling process, and for example change single values (such as block height or width) or set new alignments if an error is found. From this step, it is also possible to go back to the second step of the modelling process, to refine the measurements or even add new parts of the building. This way, the user can create one part of the building at a time, if details are needed. Also, a rougher initial model can be created to see an early sample of the building.
The post-modelling part of the system lets the user set the camera to the transformation used in specified images, which can then be used as background to a wireframe version of the model.
Texturing
The texturing process from the users point works by finding corners of the surfaces which are wanted as texture images. After specifying the same four corners in a number of images (1-N), the user lets the application work in the following way:
For each texture image pixel (x,y) coordinate:
- Use the specified corners and specified texture size to calculate the homography from image to texture.
- Use the homography to find the pixel colour value (0-255) in image i, and put the value in a histogram together with all corresponding coordinates from the specified images.
- Find the histogram bin with the highest occurrence, and use this to set the textures corresponding pixel colour value.
- If the resulting texture image gives an unsatisfactory result, remove or add more images and go back to step one. The result might be unsatisfactory due to for example partial occlusion, image artefacts (if using too few images), pixelated regions (due to perspective distortion in the original image, or the texture size being too small comparing to how close the camera gets to the finished model) or a too blurry image.
Thursday, August 31, 2006
Texture creation from multiple photos
- Get the homographies between different views from point correspondences - where the simplest method uses four clicks in the corners of the wanted texture. Using only four point might give a lower accuracy on the homography, but gives good enough results for an architectural scene, where you don't go too close to the buildings.
- For each pixel in the wanted texture:
- Find corresponding coordinate in in each clicked image (using the homographies).
- Put the pixel values for the coordinate in a histogram.
- Find the maximum occurence value in the histogram, thereby determining the value of the texture coordinate.
Reconstruction plans
I'll start by creating an interface where people can click on two photos, to use corresponding points for reconstructing a building. In the background, I'll be using some of the stuff I mentioned in the previous post, to find the movements of the camera.
To find the camera position and rotation, I'll be using RANSAC or the 8-point algorithm to get the Fundamental Matrix (F), and then use the Intrinsic camera parameters to get the Essential matrix (E). From this, the rotation and translation of the cameras will be derived.
The reconstruction of 3D points depends on prior scene and camera knowledge. If the camera parameters hadn't been known from calibration, the reconstruction could only be known up to an unknown projective transformation of the environment. The intrinsic parameters gives us the possibility to reconstruct up to an unknown scaling factor, while an unambiguous reconstruction can be done if both intrinsic and extrinsic are known (for example if you have architectural plans available).
In the situation with calibrated cameras, but unknown scene parameters (such as 3D point locations, scale, rotation etc.), we don't know the baseline of the system and can therefore not recover the true scale of the viewed scene. If we know the distance between two points in the scene, we can at least recover the scaling factor to which the reconstruction is unique.
Wednesday, August 30, 2006
3D Reconstruction from Photos
I found that through OpenCV, I can use the fundamental matrix - retrieved from at least seven point matches in two photos - and take the SVD (Singular Value Decomposition) on that as a step to find rotation and translation of points in the photos. Since I didn't at first find the SVD in OpenCV, I clumsily assumed that it wasn't in the library. After a day, I accidentaly came across it, but in what seems to be a slower version - cvmSVD() - instead of what I just found, the cvSVD() function :-)
I'll now find the fundamental matrix from point matches, use the internal camera parameters to normalize it into the Essential Matrix, and then use SVD to find the rotation and translation...
We'll see how it works - at least it gave me hope to be done in two weeks, like I had planned to from start :-)
Tuesday, May 16, 2006
Augmented Reality
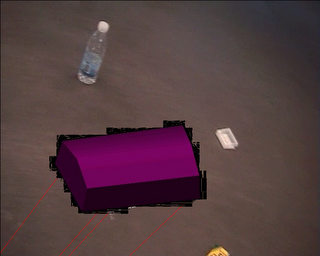
I basically started again from nothing at the start of last week, worked 6-10 hours a day and came up with this. It's great progress for just a week. This week I'll make a new part of the program, after "resting" from it (actually doing my study job) for two days. The new part will be a camera calibrator, where a user can click a few times on an image, write some measurements, and then get the camera parameters for it.
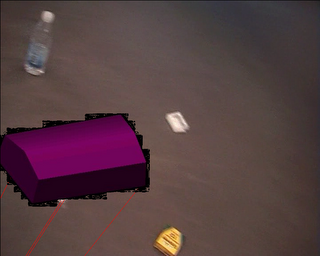